The first DeepCube scientific paper “Self-Supervised Contrastive Learning for Volcanic Unrest Detection” is published in IEEE Geoscience and Remote Sensing Letters.
Our team conducting research on volcanic unrest detection and alerting, introduces a self-supervised learning framework for volcanic activity detection globally.
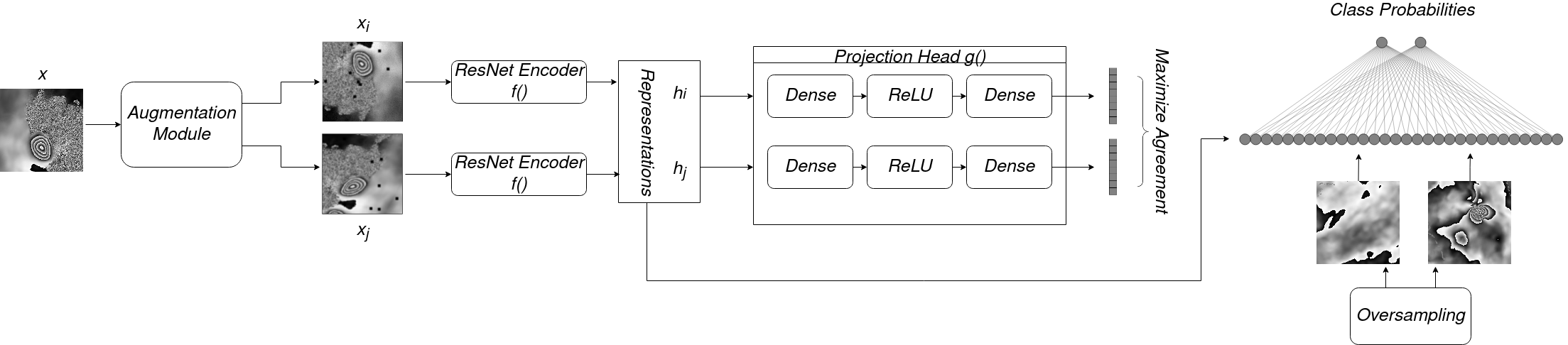
We show that our self-supervised pipeline achieves higher accuracy with respect to the state-of-the-art methods and shows excellent generalization even for out-of-distribution test data. We showcase the effectiveness of our approach for detecting the unrest episodes preceding the recent Icelandic Fagradalsfjall volcanic eruption.

To access and download the publication visit https://ieeexplore.ieee.org/document/9517282
Cite the publication: N. I. Bountos, I. Papoutsis, D. Michail and N. Anantrasirichai, “Self-Supervised Contrastive Learning for Volcanic Unrest Detection,” in IEEE Geoscience and Remote Sensing Letters, doi: 10.1109/LGRS.2021.3104506.